News
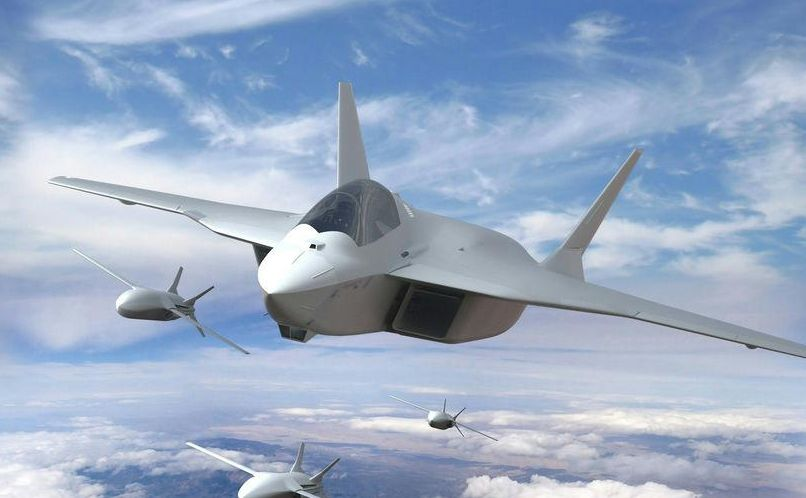
DOSSIER: SUMMARY OF THE 3AF STRUCTURES COMMISSION'S SCIENTIFIC AND TECHNICAL DAY
Article published in Lettre 3AF N°1-2024
Application to Aerospace Structures of Recent Advances in Artificial Intelligence and Data Science - Duplex SAFRAN Tech (Palaiseau) - AIRBUS SAS (Toulouse) - April 25, 2023
By Stéphane Grihon (Airbus), Bruno Mahieux (SAFRAN) and Éric Deletombe (ONERA)
OBJECTIVES
Since the advent of the "Big Data" phenomenon, it has become commonplace to declare that our data is an under-exploited mine. How does this apply to the lifecycle of aerospace structures? Indeed, the life cycle of aerospace structures is an undoubted field of application for Data Science, since an aerospace structure can be approached as a complex system which, at different scales, requires analysis methods based on either simulation or empirical methods, generating massive quantities of data. In addition, the need to monitor the quality and operational maintenance of each structure leads to a continuous flow of data from manufacturing and operations. All the elements are therefore in place to provide a fruitful application context for data science.
In the design phase, the periodic nature and high reuse rate of structures for aircraft and derived space systems (e.g. mass variants) means that data-driven models can be prepared to speed up design calculations right through to certification, and reduce cycle times and time-to-market. Traditional approaches to calibrating models on an experimental basis can also be handled advantageously through the use of data assimilation techniques using meta-models at the interface between simulation and experiment. The recent rise of physically informed models based essentially on artificial neural networks is a promising way of accelerating structural simulations, and lends itself well to hybrid simulation/experimental approaches. These two levers make it possible to improve the representativeness of models and reduce conservatism without degrading safety levels. However, there is an imbalance to be managed between experimental data, which is more costly to obtain and rarer, and simulation data, which is easier to generate and more plentiful. In any case, frugal methods requiring little data remain a priority to minimize both simulation and experimental effort.
Finally, design, repair and maintenance solutions are often to be found in the history of data yet to be analyzed. Here again, cycle times and customer response times can be reduced. Analysis of operational databases enables us to refine design conditions and adapt certification requirements, resulting in a more optimized product that retains all safety requirements. As for operational monitoring, it enables modeling assumptions to be refined and maintenance programs to be adapted to the needs of each operator, potentially individualized for each system, bringing a significant gain in operational use. It should be noted that in the context of continuous monitoring of structural health, models need to be enriched to take account of changes in properties and performance (augmented learning), enabling both diagnosis and prognosis.
All these topics are examples of emerging Data Science use cases at most aerospace designers and operators, with the support of research laboratories. They testify to the vitality of the field, which this 3AF day will explore, providing the necessary critical insight into their potential and maturity, and outlining the prospects for development in the short and medium term.
The day's plenary lecture, entitled "Data and physics-augmented intelligence for new predictive approaches", was given by Pierre Ladevèze (LMPS Paris-Saclay) and Francesco Chinesta (Arts & Métiers Paris). The aim was to put data science back into the context of engineering simulation, give examples of applications and make a few recommendations.
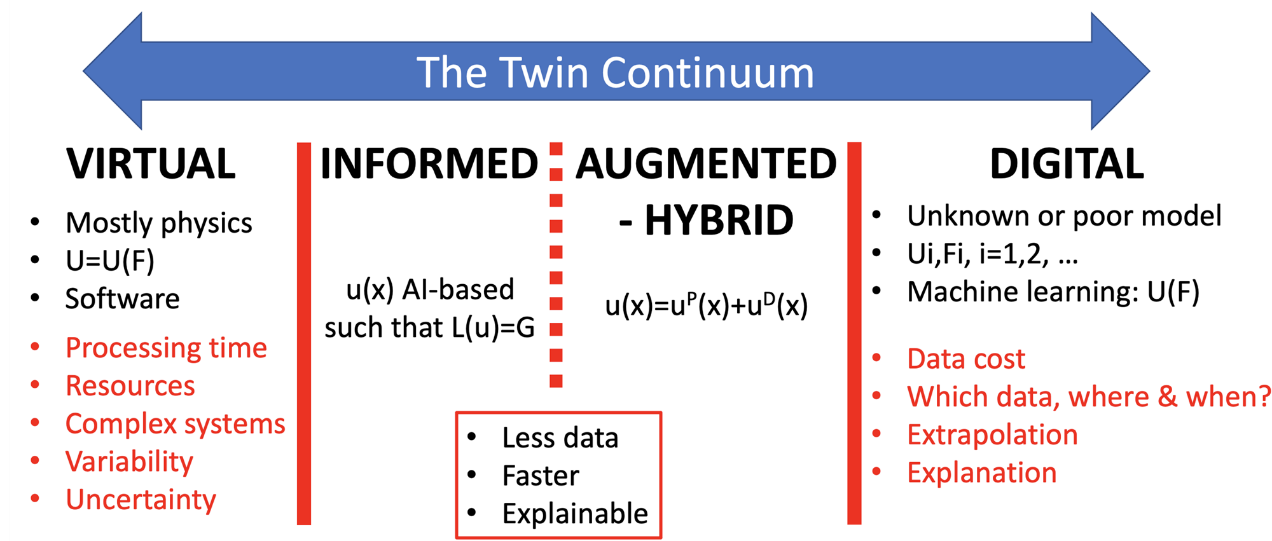
Fig.1 The current context of simulation integrating the contributions of data sciences
The context was first introduced by pointing out the clear orientation of simulation for engineering in the 21st century, from end to end, covering the lifecycle from design to operation, from component to system of systems, thanks precisely to data science and broken down according to the share of data use into 4 fields: physical, physically informed, hybrid and purely data-driven simulation.
The various analysis/processing methods were then described, and the diversity of data typologies (lists, images, graphs, curves, time series) based on simulation or measurements recalled, underlining the importance of the representativeness and completeness of these data. This was followed by a discussion of dimension reduction techniques, in particular auto encoders, which can be used to identify latent variables for a model based on a high-dimensional input space. Finally, an inventory of the various machine learning models was drawn up, and encouragement was given to start with simple models such as polynomials.
For aerospace applications, with their particularly stringent safety constraints, the results of analysis/treatment are expected to respect the principles of physics and scientifically established behavioral equations (which can only be enriched by knowledge of experimental behavior): consequently, a hybrid framework - in which model construction is driven by a hybrid function mixing distance to behavioral equations and measurement - is essential, guaranteeing the relevance of purely mathematical approaches to data science.
In the application field, a case study was first discussed (bending beam) to demonstrate the difficulty of generalizing data-driven models.
It was first shown that a purely "data-driven", empirical approach, which would take us back to Galileo's time because it ignores modern knowledge of physical behavior, is clearly to be avoided. A second application, concerning the CEA's vibration platform (for seismic simulations), was then presented: the aim of the exercise is to detect and model damage in structural connections. A coarse model is used, with its own set of parameters, and fed by measurements. Modeling error and distance to data are minimized using Morozov's weighting technique (so that the modeling error is at the same level as the measurement error). Tikhonov regularization is used. Control is by a Kalman filter using the first 3 natural frequencies of the platform, which inherently gives access to confidence intervals. The results obtained show that the response peaks are correctly represented. Other applications were presented in the field of identifying behavioral laws for materials, where it turns out that a data-driven approach can build models, from an empirical base, capable of giving results faithful to the data, with nevertheless - in the absence (and sometimes even in the presence) of a physical foundation - difficulties in extrapolating outside the domain used to build the model. A first example concerned a material damage law with different traction-compression behaviors, measured by fiber optics, for which different methods aimed at forcing the convexity of the energy function (linear function envelope, kernel methods, neural network with particular architecture) were compared. A second example focused on complex elasto-plastic behavior, where hidden variables had to be identified. Finally, another field of application was discussed, involving the diagnosis and prognosis of structural health: an example of a civil engineering project was presented, involving the analysis of the structural health of a bridge, for which a drone was used to compensate for the difficulty of equipping the entire structure with sensors, enabling data to be fetched and acquired "in the right place" using different means. The diagnosis is then established in real time using a neural network, in relation to the mechanical model, on the basis of a design of experiment containing millions of simulated cases of damage, enabling the drone (and its operator, equipped with virtual reality goggles to "see" like the drone) to detect pipe damage during inspection.
In conclusion, the main steps recommended for establishing a reliable, predictive, data-driven approach to mechanical analysis are recalled: (1) pose the mechanical problem, (2) question the available data: which, where, when, usefulness, accessibility, (3) draw up the state of prior knowledge, (4) propose a method for validating the method/results, and (5) assess the cost of the approach.
The second presentation of the day, entitled
"was given by Stéphane Grihon (AIRBUS SAS), the day's co-organizer. The aim of the talk was to present the methods used by Airbus to verify and validate surrogate models, particularly in the context of load/structural analysis. First of all, a reminder was given of what a surrogate model is: mathematical principle and industrial interests, the latter being multiple, the most common being that of speeding up simulation processes, by seeking to limit integration stages and human intervention. A wide range of applications were discussed: digital twins, optimization, new services, and hybridization, a new field at the forefront of research.
The internal Airbus context is characterized by advanced internal projects in terms of implementation in the field of loads/constraint analysis, and involvement in international working groups (EUROCAE WG114, SAE G34) aimed at building the future certification standard for products derived from artificial intelligence. EASA participates in WG114, but also produces its own documents (e.g. a concept paper, as a first certification document) and sets up projects on trusted AI. An internal AIRBUS working group has been set up to harmonize these various initiatives and develop an internal methods repository of its own, to meet the verification and validation requirements for certification. The 2022 activity on this point consisted in writing a first version of the report, starting with model evaluation, which should then drive data evaluation. Additional chapters were added on explainability and the link to the EASA concept paper. Parallel activities were also carried out on the AIRBUS Aero2stress pilot project.
To build a good substitution model, data must be complete and representative. Extrapolation does not work with pure data-driven models, which only allow interpolation within the limits of the design domain. Hybridization with the equations of physics, which is in its infancy and could confer the ability to extrapolate, is not always applicable. For the time being, therefore, at AIRBUS we're talking more in terms of generalization, and limiting ourselves to the perimeter of the "Operational Design Domain".
"Operational Design Domain". The most important property of a substitution model - its robustness - is to ensure that any data that could be incorrectly predicted is avoided during operation. This requires an error predictor. As the generally continuous design domain can never be known in its entirety, the characterization of this error is always probabilistic: we seek to show that it remains less than ε for a probability greater than 1 - δ (risk level), ε and δ having to be well specified at the start of the study. The generalization proposed by AIRBUS is ultimately supported by a compromise between bias, variance and stability, which are important properties (NB: but secondary to robustness).
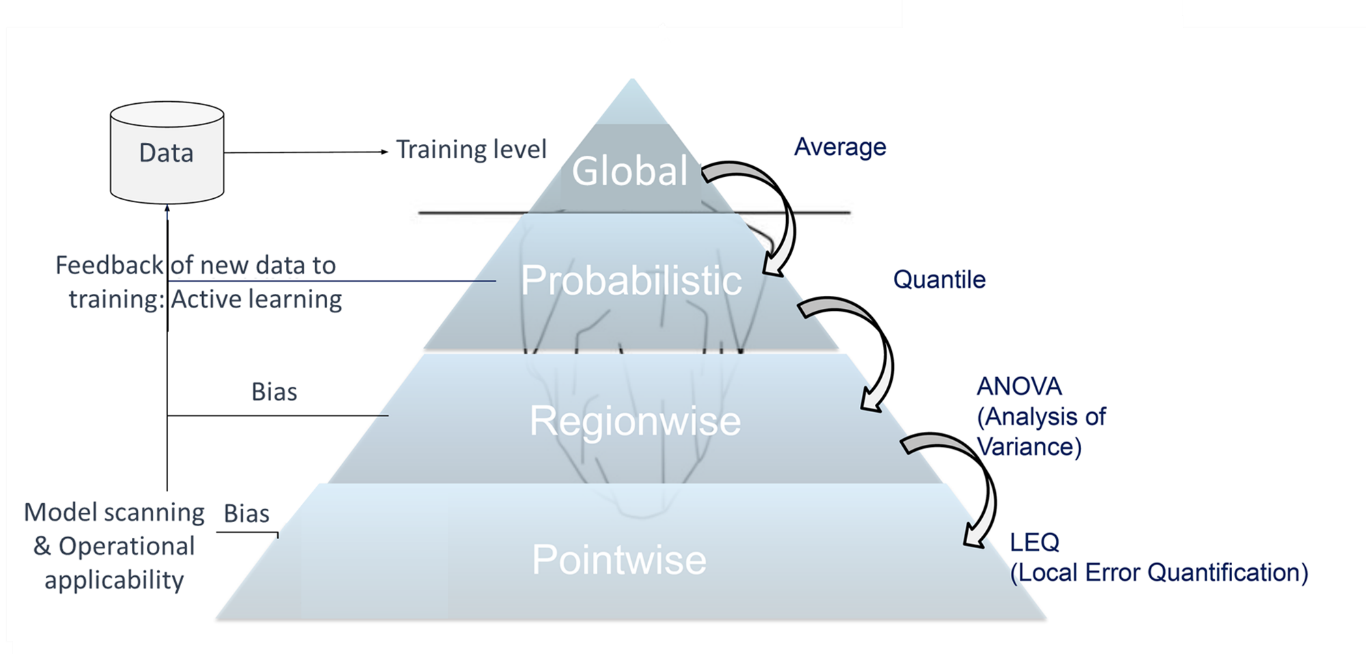
Fig.2 Pyramid approach developed at AIRBUS
for the accuracy and robustness of substitution models
The Airbus approach is, in a sense, more demanding than what appears in the standards currently being developed. It proposes a pyramid-shaped error analysis that starts from a global error level, which generally corresponds to the loss function minimized during training, then analyzes the error by region and finally by point, producing an estimator that ensures robustness in operations and must be deployed with the model. At each level of the pyramid, an iteration can occur if the error distribution is not satisfactory: either the model is called into question (hyperparameter tuning), or the data (adaptive sampling, active learning).
The "Local Error Quantification" method developed at the point stage provides a means of evaluating the error at any new point, and thus, through its use in operation, guarantees the robustness of the model. If the predicted error level is higher than specified, then the model is not used and a back-up must be employed (the original simulation, for example).
In conclusion, it is reported that the latest work carried out at AIRBUS SAS has enabled us to work on data evaluation with a critical eye, as it is an indirect verification that will be made by the quality of the model. Traceability is a major aspect of the process, given the dependence of models on data. While data verification in operations is essential, explicability is not a major point. Finally, future activities will aim to consolidate this work and apply it more specifically to the HALO (HArd Landing Optimization) project.
The third presentation of the seminar was entitled "Taking variability into account in design: industrial examples", by Frédéric Bonnet, Christophe Colette, Elisabeth Ostoja-Kuczynski, Gérald Senger (SAFRAN Helicopter Engines). Using case studies and concrete examples, the aim was to demonstrate how uncertainty is managed in relation to manufacturing (tolerancing), for helicopter engine structures. These engines remain in operation for decades, run for thousands of hours, and are in production for 30 to 40 years. They are naturally subject to variability and, what's more, to a changing environment in terms of suppliers, operators, machine tools and so on.
To explain the reasons/objectives for taking variability into account in design, the example of play resulting from a stack of parts was used (treated simply in one dimension). A first approach - the use of statistical damping - proves not to be sufficient, as it can lead to definition/manufacturing pairs that are not robust to change, for example when switching to a more precise machine that moves the design out of the original variability space: unit parts that conform to the design, yet fail to conform to the assembly after relaxation of the centering requirement. The second example, that of a turbine wheel with attached blades, is quite similar to the previous case study: if the inter-blade clearance is too high, there is a performance problem, and if it is too low, mountability is affected. A compromise must therefore be found: to this end, the possibility of having non-uniform blades and distances widens the design domain.
A multi-dimensional example (on two variables) was then discussed, concerning the way in which variability can be taken into account in design: the possibility offered by a new machine of satisfying a manufacturing tolerance interval more rigorously had an impact on a functional issue, as the most obvious solution to the problem (re-centering the tolerance interval) proved unfeasible: this illustrated the importance/necessity of taking correlations and restrictions between variables into account in a multi-variable problem. It was then explained that theoretical approaches or those based on numerical solvers should be used with caution: before doing so, the right parameters and their distribution had to be identified beforehand, otherwise misinterpretation or erroneous results could be obtained. For example, the "centered normal" hypothesis could give results far removed from those obtained with a real, observed distribution. Another industrial example, that of broaching turbine cells, was presented, for which the variability of certain parameters was evolving over time (different short-term and long-term standard deviations), due to wear and tear on the broaching tool: the design approach had to be reviewed because the optima were shifted due to the taking into account of these uncertainties, a reliability criterion interfering with a performance criterion.
In the end, we were reminded that all sources of variability had to be taken into account if we wanted a relevant result (materials, manufacturing, assembly, use, measurements, etc.), and that collecting all the necessary data meant implementing a digital twin (objective for Factory 4.0). In terms of variability characterization and exploitation, this digital twin provides a "vital map" of parts, by collecting 3 types of data: product dimensions, temporal variation in manufacturing process parameters, and context (tool no., machine settings, production batch, etc.). All this, without forgetting the major issues for the implementation of a digital twin, namely data accessibility and cybersecurity.
Ultimately, the strategy adopted by SAFRAN Helicopters is not to supply data solely to analysts (who will be involved in specialized processing, such as machine learning), but to all the players concerned, by providing extensive training in the use of basic data collection and statistical analysis tools. A final example was presented, concerning the manufacture of turbine blade feet, a complex process involving the adjustment of 35 ribs, 6 wheels being used by the operator - who has long and solid experience - to adjust the wheels: artificial intelligence was developed - in the space of less than two years - so that, after a learning phase supervised by the operator, the machine could successfully make the adjustments itself. Results: significant time saved on blade production, scrap rates divided by 3, and an operator who now runs 2 machines instead of one.
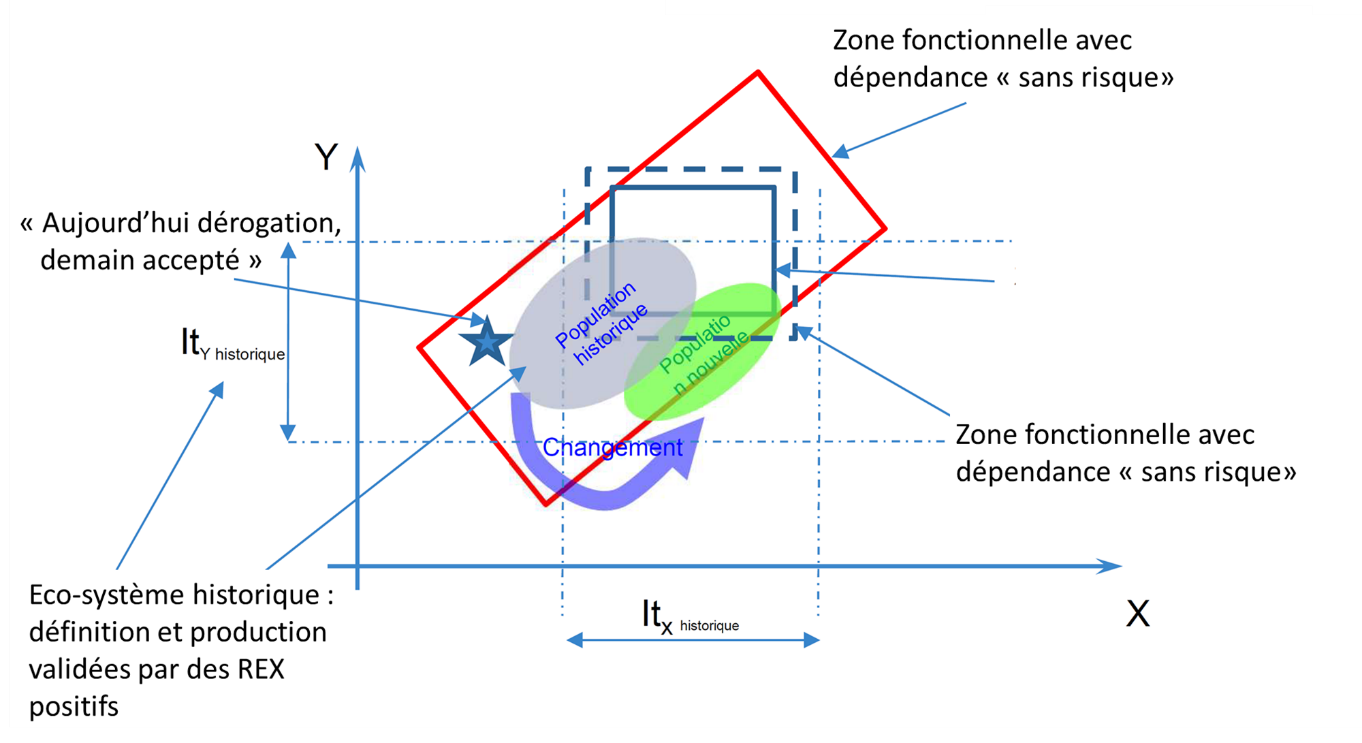
Fig.3 Taking variability into account in design at SAFRAN Helicopters
In terms of final conclusions, design and manufacturing are intrinsically linked: on the one hand, it is necessary to have a good knowledge of the means of production to succeed in designing functional parts, and on the other hand, taking variability and interdependencies into account changes the way we design. Finally, the progress expected in the field of taking account of uncertainties in design is linked to mathematical resources and computing power, but also to a collective increase in skills in data science and statistics.
The fourth presentation of the day was entitled "Quantifying and managing uncertainties from a co-design perspective", prepared and given by P. Wojtowicz and G. Capasso (AIRBUS SAS). The aim of this talk was to present a semi-probabilistic method for managing uncertainties, which can be used to solve practical problems at the interface between the worlds of calculation, design and production. This method avoids the overly conservative "case-pirate" approaches, while sparing engineers the need to manipulate probabilities, by using deterministic equivalents.
By way of introduction, it was recalled that there are thousands of parts to be assembled to make up an aircraft structure. This is done in several stages: positioning, holding, drilling, counter-drilling... The process is tedious, time-consuming and costly, but it does ensure that the fasteners fit properly in their housings, and that the assembly holds together well with low variability. The aim here was to reduce the number of steps: no more drilling and counter-drilling during the assembly phase ("as-is": the holes are perfectly coaxial). The holes would be made during the part manufacturing phase, using a method known as "hole-to-hole" (H2H), which means that the holes will be potentially misaligned (position variability), and that the hole diameters will have to be larger than the nominal diameter to be able to absorb the variability of hole misalignments. This also implies that the distribution of forces between the various links will also be non-uniform. With this H2H approach, the worst-case strategy is unrealistic and too penalizing, all the more so as its application to the whole aircraft would mean stacking/accounting for all uncertainties (on loads, materials, temperature, etc.): a rigorous probabilistic approach, which will reduce conservatism, is therefore proposed.
The first part of the presentation dealt with the effect of geometric variability on structural performance. In the general framework, geometric misalignment must be added to the quantiles of material properties and load uncertainties. The risk of under-conservatism needs to be quantified, without treating the worst case by piling up low-probability scenarios. The semi-probabilistic approach is based on ratios between the value obtained by the probabilistic approach and the value obtained by the deterministic method. This enables margins to be taken to ensure that probabilistic criteria are met. To this end, (1) factors characterizing performance degradation are defined and used to calculate the probability of exceedance. These are generally included in the formulas, and (2) intrinsic variables (geometry) are treated. Two types of uncertainty management can then be considered, depending on whether the aim is to determine :
- Reducing factors on admissible values to obtain the right level of probability. This corresponds mathematically to quantile estimation, and industrially to overload analysis,
- the factor of abatement and the variability of inputs to obtain the right level of probability. This corresponds mathematically to reliability-based optimization, and industrially to tolerance-based optimization.
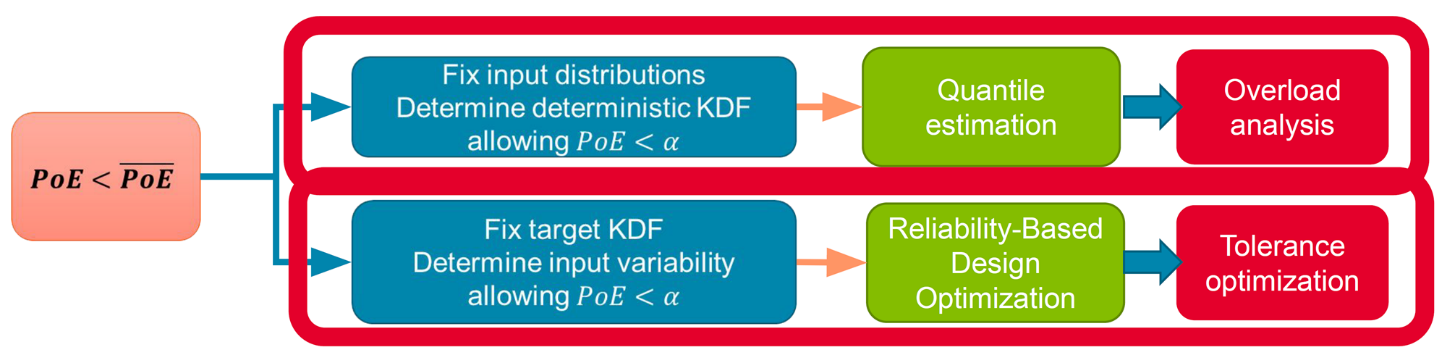
Fig.4 Two fundamental cases of uncertainty management in structural design
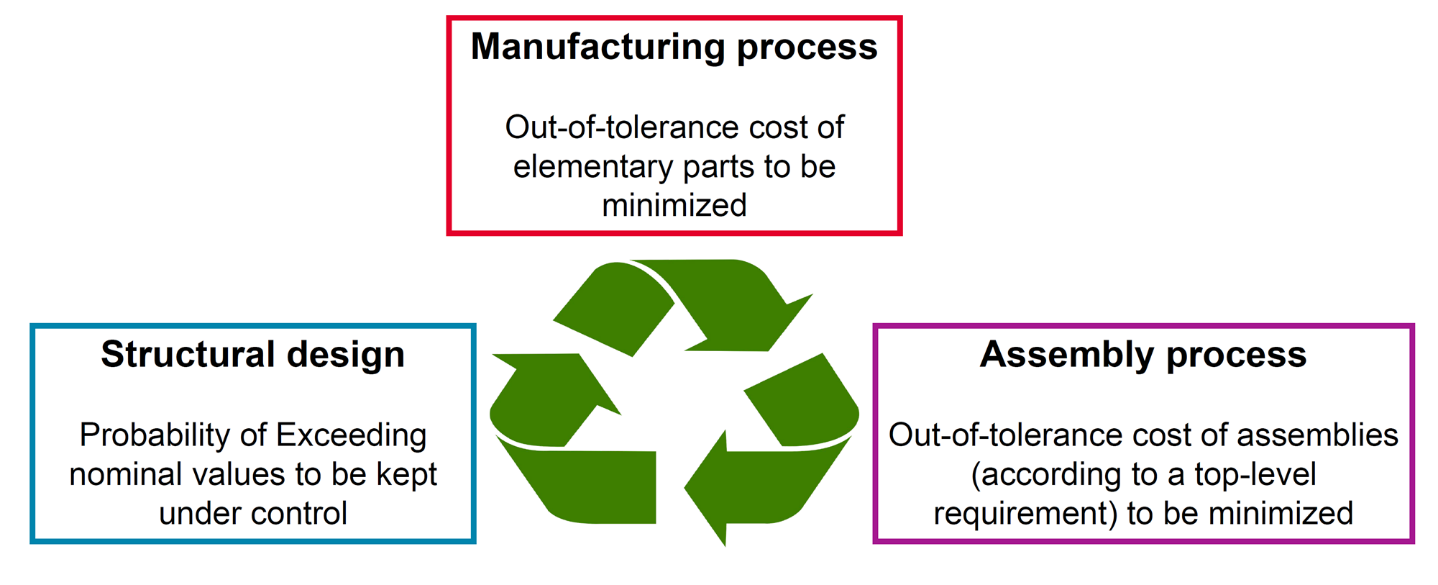
Fig.5 Proposed statistical framework for co-design (AIRBUS)
In the first case, the result is a significant reduction in overloading, particularly for more complex assemblies. In the second case, this addresses the structural design (no exceeding admissible values), the manufacturing process (reducing the cost of out-of-tolerance parts), and the assembly process (reducing the cost of out-of-tolerance assemblies). Finally, we formulate a bi-objective problem with one cost function linked to manufacturing and another to assembly, and take into account a constraint on the probability of overruns (reliability). Tolerance intervals for manufacturing and assembly have antagonistic effects, so the right compromise has to be found. Catalogs can be provided that are not perfect solutions, but satisfy the "stress" optimization constraints in semi-probabilistic terms.
Optimization results can be used to set truncation limits for misalignments, for updating by the tolerancing team. These truncation limits ensure that mechanical stresses are met. Abacuses are used to find the right compromise in terms of degradation of structural performance, and reduction in quality requirements for individual parts or assemblies.
In terms of conclusions and prospects, a semi-probabilistic framework has been set up to study the static strength of aircraft structures. A co-design approach involving manufacturing, tolerancing and load (stress) has been demonstrated: it takes the form of abacuses of proposed overload factors and catalogs of tolerance solutions satisfying mechanical "stress" requirements. A 2-level dictionary has been created, either associating the level of geometric variability with structural performance (overload factor), or the level of structural performance with geometric variability (tolerance constraint optimization). The prospects for H2H applications are numerous:
extension to fatigue, extension to composites, generalization of coupons to real aeronautical structures, industrialization of the process. Beyond H2H, the aim is to apply the approach to other design problems, to introduce the notion of probabilistic design and to support the uncertainty management deployment roadmap.
The fifth presentation of the day, entitled "In-service airworthiness monitoring for maintenance planning", was given by Mickaël Duval (DGA TA). The aim was to present how DGA, as an armaments operator, is gradually introducing data science as a tool for monitoring fleets and adapting maintenance programs to actual aircraft use. The objectives of the work carried out by DGA, which supports the armed forces' aircraft, are to improve operational knowledge, enhance flight safety, improve fleet management, optimize maintenance steps and increase aircraft service life.
When it comes to fatigue monitoring, military aviation has its own specificities compared to civil aviation, as mission profiles are very different: there are many parameters with sources of dispersion, in particular external loads due to cargo, and operational use is different. This was illustrated in the case of helicopters, where the objectives remain the same, but with the added complexity of high-frequency dynamic loads on rotating machines. Artificial intelligence potentially offers the means to achieve these objectives, by introducing the systematic use of flight data for individualized monitoring of each aircraft.
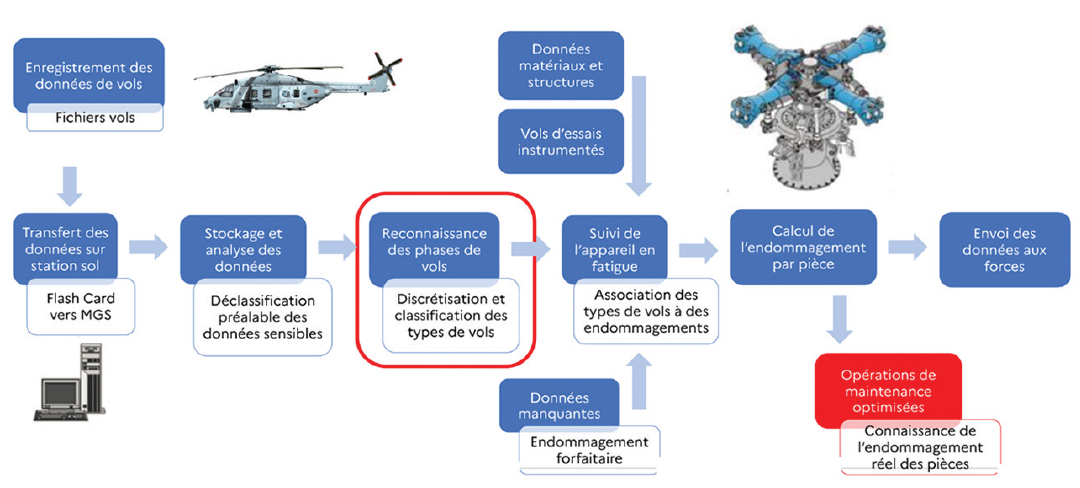
Fig.6 Principle of helicopter fatigue monitoring (DGA TA)
AI is thus used to classify the different phases of flight and associate a pre-calculated lump-sum damage to each of them. A technique combining a variational autoencoder neural network with k-neighbors is used to implement a classifier. The advantage of this approach is that it can compensate for missing data, or even predict damage in the absence of models, as is the case with some older aircraft (e.g. Canadair). One of the prospects of the work presented here concerns the reconstruction of local stress and strain spectra for more accurate damage calculations.
The sixth presentation of the day was given by Michele Colombo (AIRBUS SAS), and was entitled "Prediction of the dynamic aeroelasticity of a HALE gust using a graph neural network". Work reported on numerous occasions testifies to the considerable interest of the aerospace loads/structures community in the construction of alternative models (Multidisciplinary Design Optimization, Multi-Physics Computations) relying mostly on data-only approaches. The general framework of the presentation was that of a more marginal use of AI for learning dynamic systems, based on methods that rely not only on data, but also on knowledge of the physical laws governing their responses. Among learning methods, graph neural networks (GNNs) aim to describe systems made up of interrelated blocks, and examples of GNN applications for learning dynamic systems have recently been published (DeepMind, 2018, mass systems; Lemos, 2022, orbital systems). The aim of the work presented was to apply the technique to the learning of an aircraft aeroelastic model, with the eventual idea of using it to take measurements into account and establish the link with all measurements made in flight.
In a graphed neural network, information is encapsulated in the form of variables associated with the edge, node or global context (quantities v, E, U in Fig. 2). Organized around an encoder, a processing core and a decoder, GNN makes it possible to work on a latent space (a more relevant representation space than the space of initial variables). In the case presented, the physical variables of the aeroelasticity problem were assigned to the input variables as follows [Nodes(V) : Node positions, velocities, masses; Edges(E) : Structural characteristics; Global(U) : aircraft states and gust wind] and outputs [Nodes(V'): speeds at t+1; Edges(E'): loads at time t; Outputs(U'): derivatives] of the graphs, allowing rapid substitution of aircraft loads and aircraft dynamics.
Each block contains a physical operator, with the possibility of symbolic identification to discover the mathematical nature of the interactions. The original code (from the literature) was adapted to aeroelastic specificities. Linear aggregators were added to cover the need for a theoretical ability to observe aircraft shape before load calculation. A dedicated encoding, processing and decoding structure was developed. Tests on a toy model, then on Deep Mind's cas-test were carried out, with better results and good long-term predictive capability. The work was then transferred to a HALE (High Altitude Long Endurance) UAV (Unmanned Aerial Vehicle): the SHARPy HALE longitudinal burst university case (1-cos). Sixty simulations were carried out to learn and validate the model, with 20% learning/test partitioning over the time interval. Very simple neural networks of 128 fully-connected 2-layer neurons were used. A "long term loss" approach was adopted for learning regularization, which in the end took just 30 minutes, with inference being almost immediate. The approximation results were found to be very good.
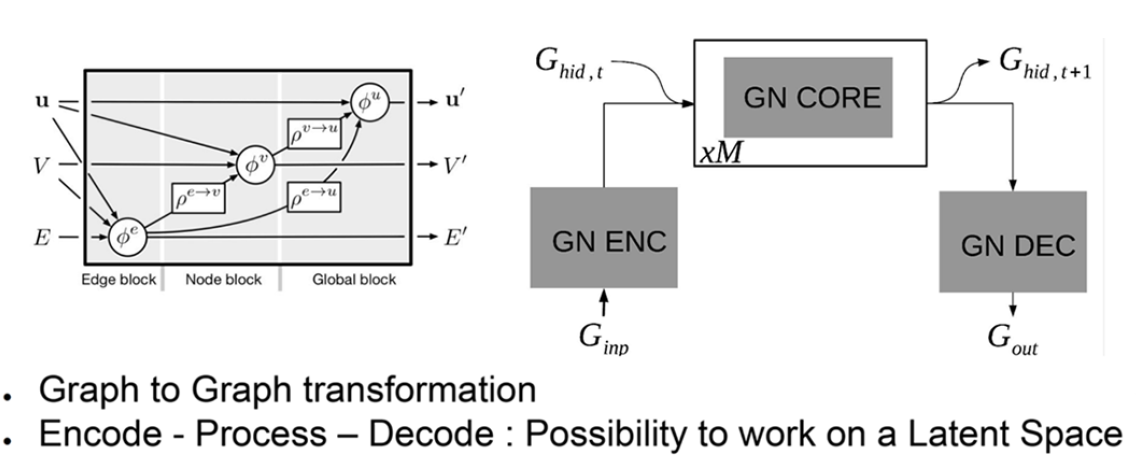
Fig.7 Architecture of graph neural networks (AIRBUS)
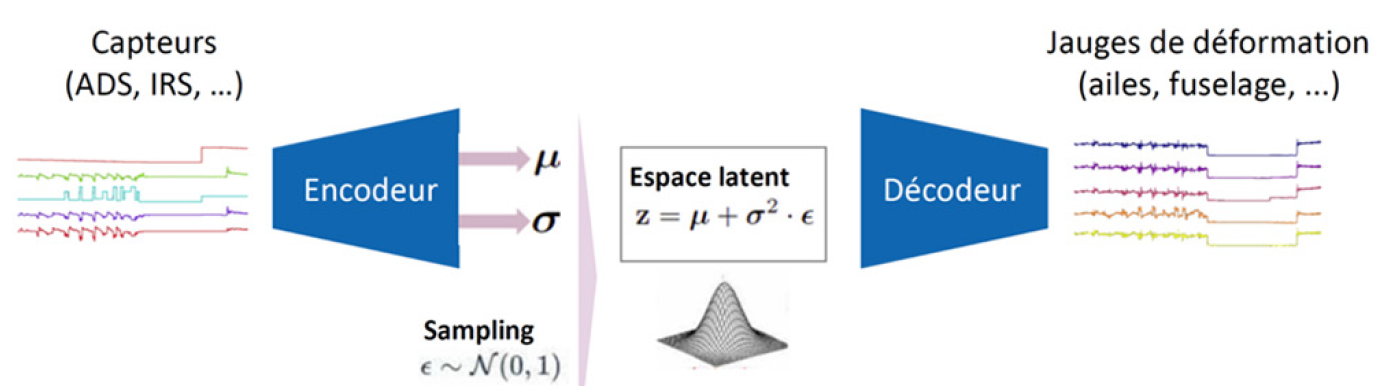
Fig.8 Architecture chosen by the winner of the Dassault-Aviation challenge
In conclusion, the work presented concerned the adaptation of GNNs to problems of aeroelasticity, an adaptation which led to good results on load calculations. With a "long-term loss" approach implemented for aeroelastic dynamics, the results were obtained in autoregressive mode, with the well-known limitations of multi-layer networks taken as universal approximators. Possible improvements include replacing the neural network with neural ODEs (Ordinary Differential Equations), the use of symbolic identification, and comparison with a real system or other simulations. Concerning neural ODEs, the use of an ODE solver on a function modeled with a neural network is envisaged: the adaptation of NODEs to the graph case with a control input has already been explored with a split between static and dynamic graphs, and has given good preliminary results.
The seventh presentation of the day, entitled "Virtual instrumentation of customer aircraft through learning", was given by Stéphane Nachar (DASSAULT AVIATION). His objective was to present how Dassault Aviation is developing a system enabling it to calculate the actual damage to customer aircraft structures based on operational measurements. Since the 2017 3AF day "Big Data and its application opportunities in the field of business aircraft aerostructure", data science at Dassault Aviation has been deployed with multiple applications linked to better knowledge of usage statistics, sizing criteria, vibratory environment, climatic impact... Collaborations were initiated through theses on vibratory environment and failure prognosis.
The subject of the presentation was virtual instrumentation for business jets, aimed at eliminating the need to install physical gauges on aircraft, by simulating them using machine learning models, with the models trained on test data from equipped aircraft (booms, gauges, etc.). The aim is to predict stress and strain states at specific points of interest, based on flight parameters measured by functional sensors (ADS, IRS, control surfaces, etc.).
In this context, Dassault Aviation proposed a challenge to over 35 candidates, which was won by a consortium comprising start-up Aquila Data Enabler and ISAE SupMeca. Their learning solution was based on a classic analysis scheme: dataset creation, anomaly elimination, regression, validation, model deployment. Temporal data were used, and sensor failures had to be detected. A dynamic dashboard was used for validation by business experts. The architecture chosen was based on a variational autoencoder (VAE). A neural network was used, as it was better suited to multivariate time-series dynamics and anomaly detection.
Knowing that the model would generalize poorly on points containing anomalies, these points had to be discarded. Two types of metrics were used for generalization: reconstruction error and likelihood of latent vectors. Anomalies can be distinguished by 2D visualizations (principal component projection or t-SNE): anomalies appear as distant clusters in latent space. In addition, the uncertainty of predictions can be quantified using quantile regression, giving prediction confidence intervals. Finally, a dashboard was used to assess the quality of the results: errors, according to different metrics, presented by flight. The results turned out to be very good, and often better than the results of physical models. In addition to prediction, the model could also be used to detect anomalies (or points of surprise) using a normality score, based on the probability of reconstruction in latent space. The correlation between different sensor families could also be analyzed.
To sum up, a model has been developed that is suitable for both prediction and anomaly detection. It can predict the deformation cycles of the device, associated with confidence intervals. Latent space analysis can provide an additional form of explicability. The model is suitable for hybridization with physics (work in progress on ODEs). Inference is instantaneous and there is little learning curve. Aquila offers general frameworks to help build these models.
In terms of conclusions and prospects, the predictions were obtained with sufficient accuracy. The next stage will focus on damage calculations using conventional simulation tools. Other possible uses for virtual sensors include improved monitoring of domain opening flights, detection of anomalies in the measurement or acquisition chain, and detection of early signs of system failure. Other potential applications include hybridization between physical models and models learned from data, or frugal learning applied to turbulence model generation, generative CAD, etc.
The eighth and penultimate presentation of the day was entitled "Conception de la structure d'une automobile par simulation 3D, apport de la data science", by Yves Tourbier (RENAULT). The aim of the presentation was to explain how Renault uses data science to build the most frugal models possible to conduct its automotive crash design optimization studies with the minimum of analysis. The work presented involved numerical optimization and model reduction using data science. They were developed and tested mainly in crash design, but also in acoustics, combustion and vehicle aerodynamics.
A constraint imposed on the vehicle design optimization exercise is that it must use the same model as in simulation (design, validation): a simplified model can be used to seek the solution, but it will be necessary to converge or at least verify with the complete model that the desired results are achieved. The parameters to be considered are thicknesses, part materials, shapes, number and position of welding points, presence/absence of reinforcements: there are several dozen for each case study. To deal with its optimization problems, RENAULT uses the design of experiments method, with a cost in terms of number of 3D simulations of 3 to 10 times the number of parameters, and study times of 2 to 4 weeks. The more automatic methods tested, such as EGO (Efficient Global Optimization), give costs of 10 to 20 simulations per parameter, which is too heavy: hence the search for a new optimization method (where the number of crash calculations would be close to the number of design parameters) which would reduce the cost and time of optimization studies to cope with the evolution of models (increasingly large), demand for optimization, and the expansion of studies in number of parameters.
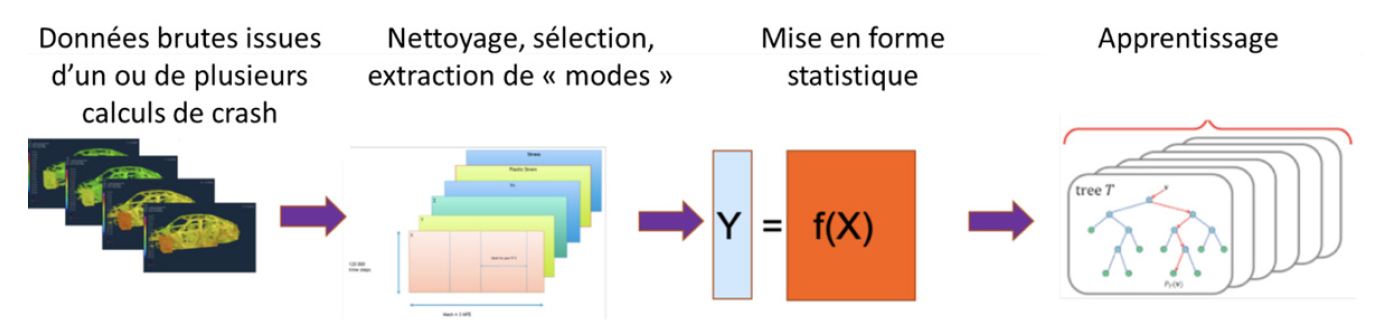
Fig.9 Principle of the ReCUR method used at RENAULT
A few figures: a crash model involves 6 to 10 million finite elements, 120,000 to 200,000 time steps, 20 observables per node (20 fields). In practice, we work on 200 time steps and restrict the fields to points of interest. Research avenues aimed at reducing individual computational costs, having a reduced intrusive model, taking advantage of past studies (transfer learning) or using multi-fidelity models are considered difficult. The Régression-CUR method, a non-intrusive reduced model taking advantage of the particular definition of the parameters, was preferred. The first step is to separate the time and space variables for each field, as is generally the case with ROM methods (e.g. POD), but Regression-CUR requires a reduction method that can be traced back to the design parameters. For this purpose, the EIM method has been chosen, as it selects time steps and mesh nodes, enabling the design parameters to be traced. The techniques are the same as those used in conventional ROM methods: each field M is decomposed by M=CUR with R the matrix of temporal modes, C the matrix of spatial modes and U the matrix of coefficients. These modes are then used to construct a regression problem. Each mode or pair of time x space modes corresponds to a column in the regression matrix X. This is the feature construction part. The Random Forest method is then used to predict the Y response.
The principle behind the construction of X columns is based on the mixing of optimization parameters and field parameters, taking advantage of the fact that parameters have a global definition (the thickness of a part) but also a local one (the nodes of the part mesh inherit the thickness of that part). As modes are field selections, they also inherit design parameter values. This makes it possible to integrate both field-parameter simulation and design-parameter optimization into the regression model. Additional parameters can be added that are not linked to mesh nodes, such as weld points. The notion of node reduces the number of parameters: from a set of part thickness parameters to a single mesh node thickness parameter. However, there may be discontinuities in predictions, as nearby nodes may belong to different parts.
An example of a rear-impact study with criteria control on the tank was presented. The idea was to correct unsatisfied criteria with a minimum mass penalty. NB: the crash calculation is not perfectly repeatable (instabilities in the physical calculation such as buckling, or purely numerical). Two reduced models were used, one for nominal (on average), the other for dispersion.
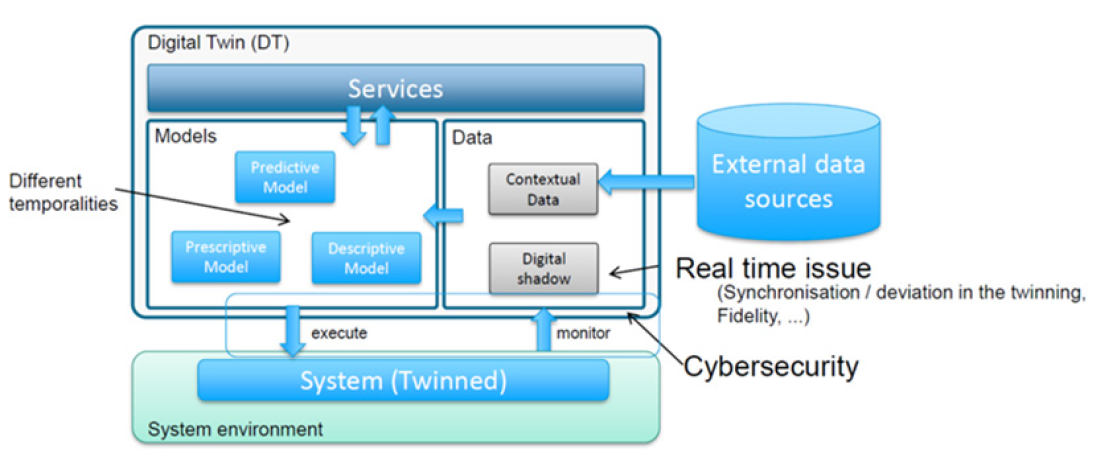
Fig.10 Schematic diagram of the digital twin (see document IRT-SystemX/JNI3)
Optimization was carried out in two stages, and a satisfactory solution was finally obtained, with a limited addition of 4kg.
In terms of conclusions and prospects, the ReCUR method presented enables industrial studies to be carried out at lower cost. The tool is currently being industrialized by ESI (code PAMCRASH). DEIM (Empirical Interpolation Method) is not so well suited to crash studies (too many modes). An evolution towards neural networks is envisaged, as well as the integration of additional constraints, such as conservation of total energy (PINN), combined with Transfer Learning to exploit the results of previous studies.
The final presentation of the day, entitled "Building the first steps towards the digital twin of tomorrow's engine", was given by Dohy Hong (SAFRAN Tech). The aim of the presentation was to explain what the digital twin is for SAFRAN Tech, and what the main challenges are, bearing in mind that the primary objective is to predict the ageing of structures over time, but also to improve the sizing of these structures with regard to the new ecological challenges.
An overview of the problem at SAFRAN-Tech was first presented: the digital twin is based on a numerical model, and can be applied in 3 ways: (1) combined use of machine learning and physical models, (2) data fusion mixing experimental and simulation data, and model mixing, or (3) physically informed approaches, taking into account geometric description, equations but also feedback to integrate correlations for example.
Its implementation requires 3 ingredients: the real system (instrumented), the digital model, and the data (from the instrumentation and the model). Depending on the context (design - production - operation), specific digital twins are built using these 3 ingredients. At SAFRAN-TECH, these digital twins must be interoperable. The digital twin offers services interacting with data and models, which are respectively monitored and operated by the real twin system. The data is processed in real time, and constitutes the digital shadow of the product. There may also be a need for data external to the system.
In concrete terms, the problem is formalized here as a Kalman filter (offering a natural framework for quantifying uncertainties) with two laws: a transition law (behavior) and a data law (measurements). The learning effort is focused on the transition law, and in addition to intuition, assumptions and physical knowledge must also be taken into account. Particle filters based on weighted sampling are also used.
The viability and operationality of the digital twin are based on 3 prerequisites: (1) digital continuity, to ensure data traceability throughout the product life cycle, (2) representativeness of the digital clone, which reproduces the functioning of the system and in particular its ageing, and (3) the ability to estimate the state of health, based on historical data. SAFRAN is focusing on this aspect.
The first technical challenge facing the digital twin object - when the targeted (short-term) application concerns the search for signatures (anomalies, breaks, trends), diagnostics (maintenance fault resolution) or prognostics (production planning) - concerns the wide variety of data sources to be processed: operating data (in continuous recording : QAR, black box equivalent), shop data, bench data, etc. The models developed are based on agnostic approaches, with machine learning, but can use physically informed approaches (PIML) to target the search, in particular the selection of variables (features) and ensure a level of interpretability. The second challenge relates to the progressive degradation of the system as a whole over a longer period of time, with the study of aging requiring representations in reduced models (e.g. temperature), given the amount of information to be stored/interpreted. Finally, individualized digital clones represent a challenge for digital continuity, and for the use of artificial intelligence: it is indeed necessary to take advantage of data at all stages, design (test bench data), reception and operations (flight and configuration data), inspection, and maintenance.
In conclusion, for SAFRAN, the digital twin is a means of better identifying the state of a system and labeling data. It enables gains in system simulation, problem resolution and maintenance cost reduction. SAFRAN is also considering opening up its data beyond its own walls: how can a digital twin be shared with external partners? Cooperation with IRT SystemX is underway to develop a common framework for building digital twins. Work on digital twins for batteries and landing gear is also being discussed.
ROUND TABLE
(moderated by Pierre Ladeveze, Francisco Chinesta and Stéphane Grihon) and day's conclusion
Proof of the topicality and liveliness of the subject, mention was made during the day of numerous industrial and research projects on the subjects covered during the session (some in international collaboration, such as with Singapore, within EUROCAE, ASME, etc.), of the CREATE campus of excellence, with hundreds of researchers and numerous industrialists involved, of a Groupement de Recherche (GDR) created in January 2023 to work on data-augmented simulation and machine learning (GAIA), and so on. With an audience of almost 80 participants, divided between the SAFRAN-Tech site in Paris and the AIRBUS-SAS site in Toulouse, a large number of general and technical questions were asked, both in the wake of the presentations and during the round table discussion that brought the day to a close, and the exchanges - which it is not possible to recount in their entirety in this summary - went well. All agreed that the very broad scope of potential applications and the power of these methods are pushing back the limits of what can be "solved", and opening the way to the treatment of new problems, which in turn will lead new designers and researchers to challenge their knowledge, as well as the most powerful emerging technological capabilities. In this respect, more in-depth thematic studies were suggested, for example on the following topics: (1) digital twin, maturity, locks, uniqueness or diversity, (2) management of uncertainty, status on industrial implementation, difficulties and solutions, (3) safety of data-based models and certification. We hope this will give rise to future exchanges between participants, in more specific ways.
THANKS
3AF would like to thank once again the speakers who - by sharing their expertise - made this scientific and technical day possible, as well as the organizers SAFRAN-TECH and AIRBUS-SAS, who welcomed the participants to their premises.
No comment
Log in to post comment. Log in.